End of the road for old-school customer analytics metrics? (Part 1)
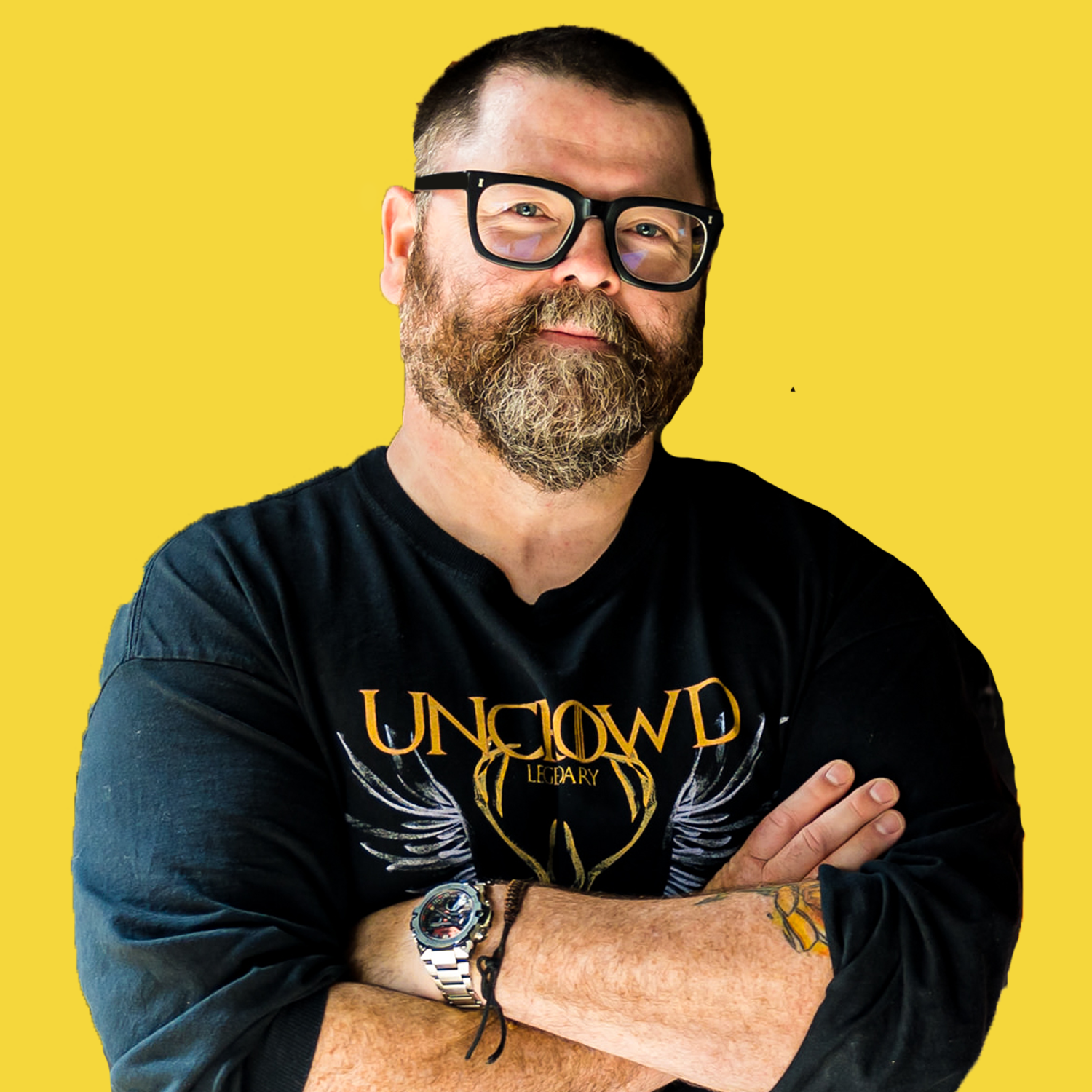
In a series of two articles, we examine the state of current CX analytics and identify the gaps in insight left by traditional metrics. And we look at a new kind of metric that can fill those gaps in our understanding of CX performance.
Old-school CX metrics leave you guessing
Your CX analytics toolbox has well-established options for building a picture of your customer experience. NPS, CSAT, CES, digital analytics, purchase behaviour metrics, footfall, churn rates; these tools generate bucketloads of data and can deliver some useful insight. But the picture they build of customer preference has gaping holes in it.
In this series, we look at some of the limitations of these old-school analytics metrics:
- Customer perception feedback
- Channel engagement metrics
- Customer lifecycle measurement
Customer perception feedback
The mainstay of customer analytics for decades, customer perception feedback relies on customers sharing what they felt about an interaction with a brand or retailer.
Net Promotor Score (NPS), Customer Satisfaction Score (CSAT), Customer Effort Score (CES) and product review analysis are perception feedback metrics.
These metrics require real-time, responsive, multichannel activation of large volumes of surveys completed ideally by identifiable consumers to ensure that samples are representative of the buyer market.
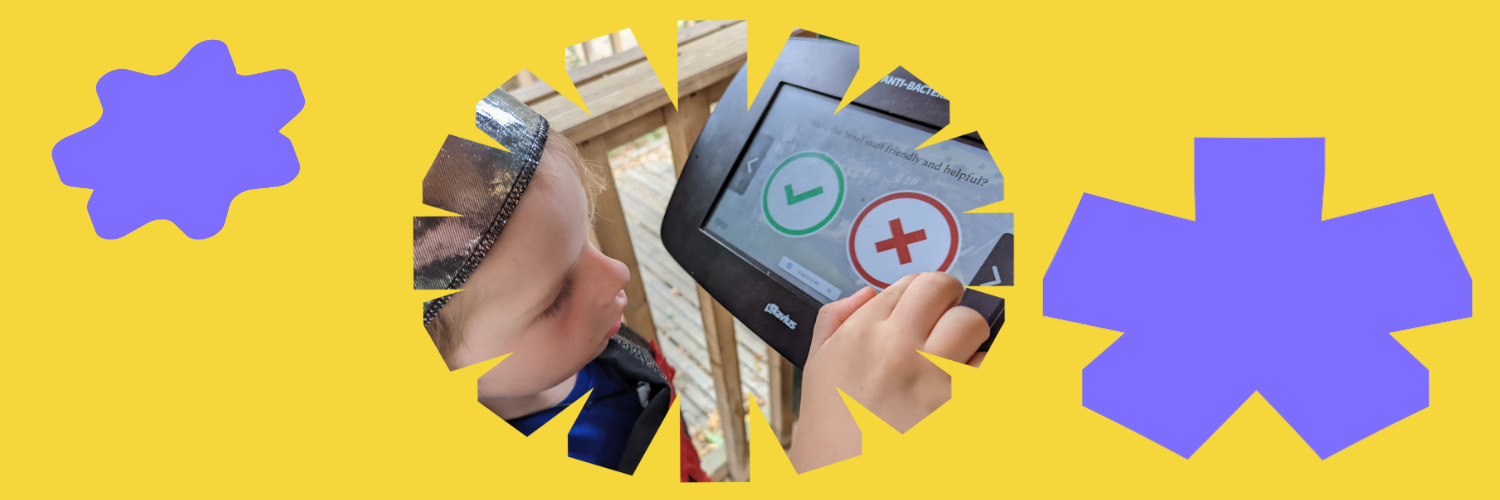
Challenges of customer perception feedback
Commerciality
The connection between perception insight and commercial application remains tentative and costly to analyse or engineer in a robust way.
Lack of actionable detail
Data aggregated for a simple score doesn’t necessarily provide the granularity required to be confident of what changes to make to improve things. Returned feedback is subject to sector and category-specific nuances, making it unreliable as a basis for a predictive model in the field.
Gamification
Customer surveys are often linked to staff bonuses, with financial rewards for high scores and negative consequences for low scores. This leads to gaming of scores by staff, for example asking customers to return high scores.
Negative bias
Engagement with customer surveys is typically dominated by the disgruntled and ignored by the indifferent or satisfied.
Input is questionable
Interpretation of the data from customer surveys doesn’t consider subjectivity. A customer may give a different response to a survey in different contexts and mindsets.
Point-in-time
Customer surveys record a customer’s opinion at one interaction point, but don’t effectively predict a customer’s long-term loyalty.
The ‘one-number’ problem
The basic structure of most customer surveys is open to bias from the affect heuristic. Surveys often ask customers to pick one number to rate their experience. But a choosing a keystone number is a system-1 thinking trigger. System-1 thinking is fast and instinctive compared to more considered logical system-2 thinking. This means the conclusions you draw will be based a non-rational decision on the part of the consumer in that moment, in other words risky and unreliable data.
Inward-looking
Customer perception insight tends to concentrate on data from within an organisation, ignoring competitive influences in market.
High data volume
For customer perception feedback to deliver meaningful insight, large volumes of data are needed. Survey engagement volumes need to be high to be representative of a potential market and often need to be from verified customers.
Next up: Channel engagement metrics
Uncrowd is on a mission to improve every customer experience on the planet through a unique combination of CX observation, quantitative measurement and comparative results. Our data is objective, empirical, and always shows your next best action.